ETH Zurich together with SBB addressed the issue in a project funded by the ETH Mobility Initiative.
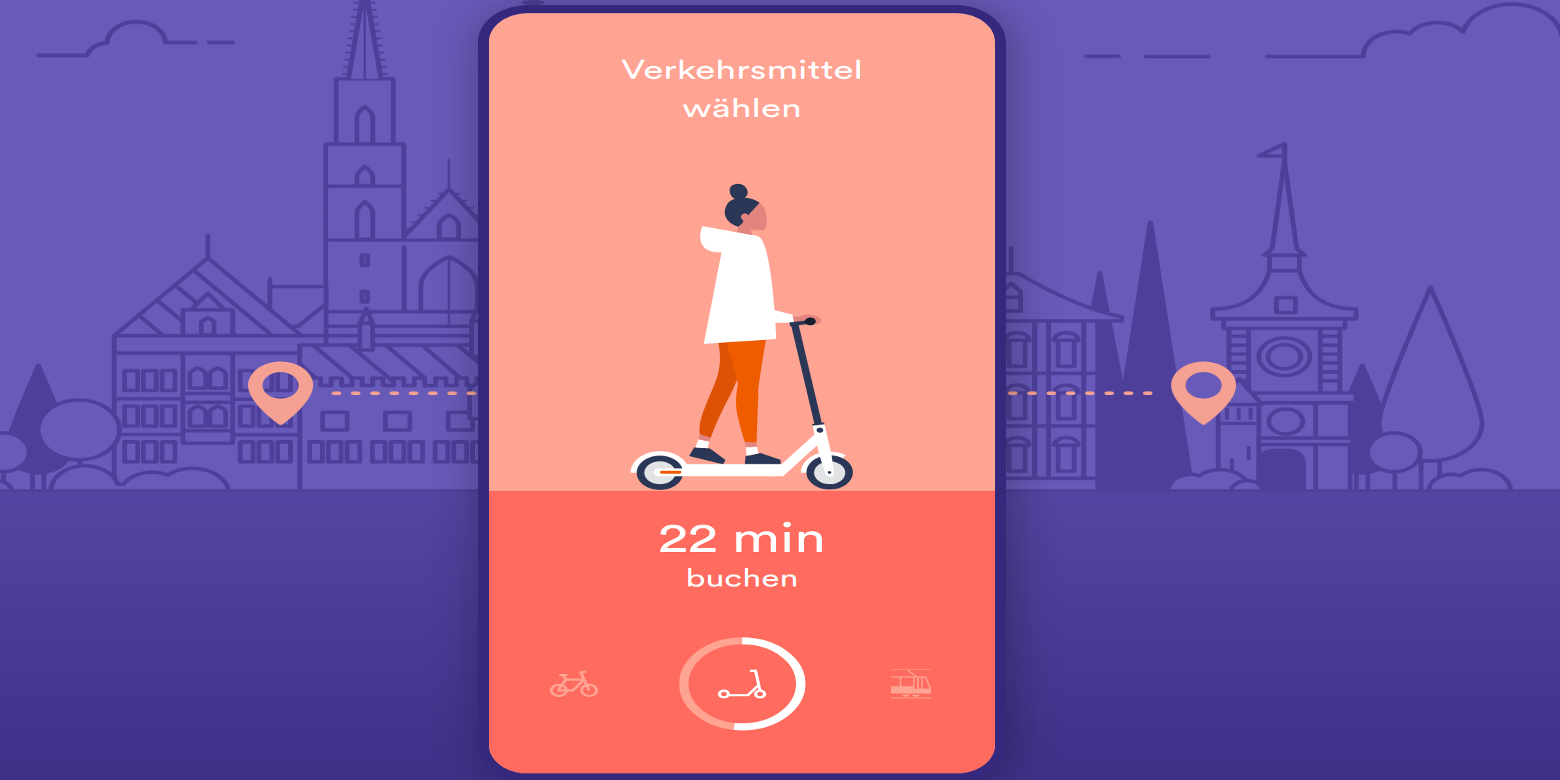
Mobility as a Service (MaaS)
Mobility as a Service, MaaS for short, focuses on the use of means of transport rather than ownership. In order to make traveling without a private car as attractive and easy as possible for customers, MaaS offers integrate travel planning, booking, and purchase for several means of transport such as e-scooters, public transport, and other sharing offers in a single app. However, due to a lack of substantial behavioral data, it remains unclear whether and how MaaS actually changes travel behavior. At the same time, collecting and processing these data becomes increasingly complex as there are a multitude of relevant tracking and context data sources available [1].
Yumuv
To investigate these questions, the Empirical use and impact analysis of MaaS (EIM) project was designed around the launch of yumuv, a new MaaS product offered by SBB and PT providers in Basel, Berne, and Zurich. Yumuv allows you to book several shared modes of transportation such as e-scooters or e-bikes via a single app and with a single registration.
Additionally, yumuv offers so-called mobility bundles that further simplify the user experience, e.g. by offering 60 minutes of mobility per month independent of the mode of transport or provider. In this offer, not only the price is reduced with respect to the real costs but also the unlocking fee (the typical fee you need to pay to start using the service on each trip).
The two main goals of the project were to investigate how bundles impact human mobility behavior and how to create machine learning applications tailored to the needs of such novel mobility applications, notably the possibility of combining many different data sources while being memory and computationally efficient.
In order to answer these questions, the team collected empirical data in a 3-month tracking study with a total of 498 participants. The result of the tracking study is the largest empirical dataset on MaaS usage that was so far recorded. The main outcomes of this study are that the available MaaS bundle jointly increased the usage of e-scooters and public transport [2] and that the effective sustainability of shared modes (especially e-scooters) strongly depends on the transport modes they replace [3]. This research project was led by Professor Martin Raubal who is head of the Geoinformation Engineering group at the Institute of Cartography and Geoinformation at ETH Zurich. The usage analysis and the sustainability of e-scooters was performed by Daniel Reck from ETH Zurich's Institute for Transport Planning and Systems, led by Professor Kay Axhausen. From the methodological and scientific point of view it was possible to demonstrate that a graph-based representation of individual mobility, consisting in representing an individual mobility profile as a graph with visited locations as nodes and the travel between locations as edges, is computationally efficient, more privacy friendly than comparable mobility profiles [4, 5, 6], and can be used in combination with existing well-known graph-based inference methods [7].
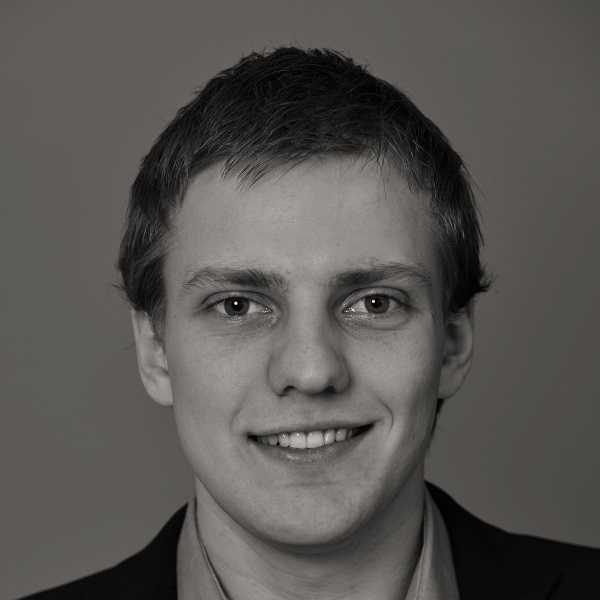
“It was very exciting to see everything come together at the launch of the project and then to see the product working in real life.”Martin Henry - Mobility Information Engineering Lab (MIE)![]()
A great mix of research and real word impact
Henry Martin is a PhD student at the Mobility Information Engineering Lab (MIE) which is part of the Chair of Geoinformation Engineering ETH Zürich. He has been working in close cooperation with SBB as part of his PhD work. He comments: “The most exciting thing was the mix of research and real-world impact. SBB and their partners were working on the app design of their new MaaS pilot product while we prepared the research project and the tracking study around this launch in parallel. It was very exciting to see everything come together at the launch of the project and then to see the product working in real life”. Despite the added complexity of synchronizing with the real product released and additional stakeholder management, the scientist looks back to a great experience: “For the processing and analysis of the data we formed a joint team with researchers from ETH Zurich and data scientists from SBB. I could really benefit from their experience to deploy preprocessing and analysis methods (something that is often overlooked in academia) but could help the SBB team to implement state-of-the-art methods.”
Successful interactions between researchers and implementation team with continuous feedback
Merlin Unterfinger, Jorim Urner, and Thomas Hettinger, Data Scientists in the unit external page Innovation, Research and Incubation, SBB AG did refer to the previous successful collaboration with the ETH team in the rather recent Green Class project back in 2017: “The yumuv project was in a way a continuation, not in terms of content, but methodologically. We were able to adapt and develop certain processing steps of the tracking study from the previous project. This is very gratifying when successful research collaborations lead to follow-up projects.”
-
Merlin Unterfinger - SBB AG -
Jorim Urner - SBB AG -
Thomas Hettinger - SBB AG
SBB also looks back to a very successful cooperation experience: “We particularly appreciated the high methodological competence of the research partners, as well as the close accompaniment throughout the entire project. In the early phases of the project, the closely timed exchange with the experts allowed us to take into account current knowledge on the subject from past and ongoing projects worldwide.”
“We chose the approach of working together directly on the SBB internal IT infrastructure. This enabled seamless collaboration and allowed us to benefit from rapid iterations with continuous feedback. In addition, the chosen setup was very strong in terms of data protection, as the data never had to leave SBB at any point and access rights were constantly under our control. Changes and enhancements of both sides, thus, got deployed into the productive data processing pipelines immediately and without further detours. We were able to process and evaluate the data (almost) in real-time during the ongoing project. Thus, we were able to continuously update the business team with valuable insights on the status and progress of the research project. Finally many insights from the research project could be directly incorporated into decisions for the further development of the product. We were also surprised by how well it worked to use tools from research that implement state-of-the-art methods (for example, the TrackIntel framework) for productive pipelines. We would like to thank our project colleagues at ETH Zurich for the good cooperation and would be happy to continue the collaboration in a future project.”
[1] Martin, H., Reck, D.J. & Raubal, M. (2021). Using information and communication technologies to facilitate mobility behaviour change and enable Mobility as a Service. GI_Forum Journal for Geographic Information Science. external page DOI
[2] Martin, H., Reck, D.J., Axhausen, K.W. & Raubal, M. (2021). ETH Mobility Initiative Project MI-01-19 Empirical use and Impact analysis of MaaS. ETH Zurich. external page DOI
[3] Reck, D.J., Martin, H. & Axhausen, K.W. (2022). Mode choice, substitution patterns and environmental impacts of shared and personal micro-mobility. Transportation Research Part D: Transport and Environment, 102, 103134. external page DOI
[4] Martin, H., Perez-Cruz, F. & Raubal, M. (2022). A graph-based representation for human mobility data. (in preparation).
[5] Martin, H., Wiedemann, N., Reck, D.J. & Raubal, M. (2022). Graph based mobility profiling. (under review).
[6] Martin, H., Wiedemann N., Suel E., Hong Y. & Xin Y. (2022). Influence of tracking duration on the privacy of individual mobility graphs. 17th International Conference on Location Based Services (LBS 2022). external page DOI
[7] Wiedemann, N., Martin, H. & Raubal, M. (2022). Unlocking social network analysis methods for studying human mobility. AGILE: GIScience Series, 3, 1-12. external page DOI