OMISM: On-board Monitoring for Integrated Systems Understanding & Management Improvement in Railway
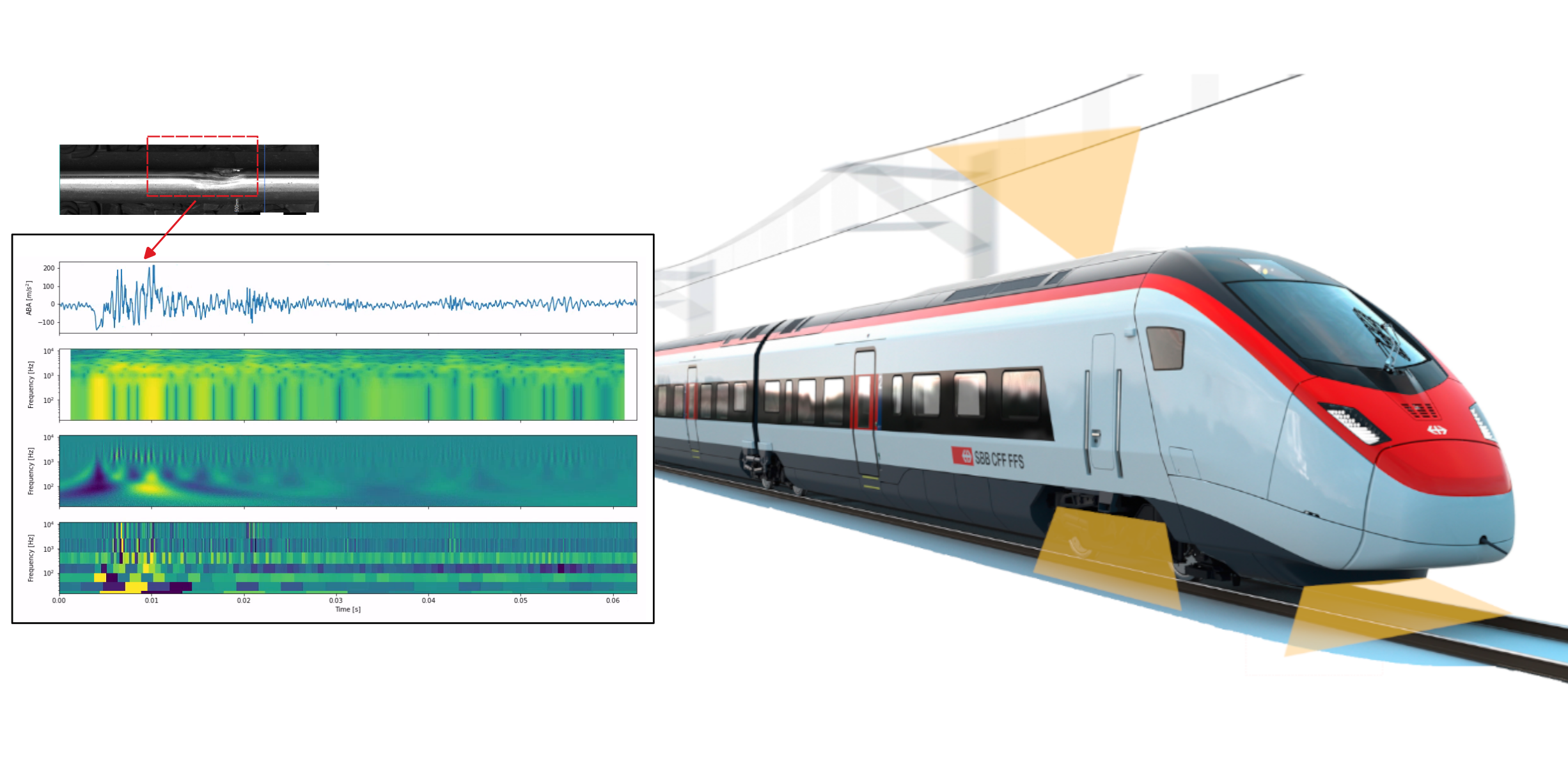
Switzerland’s railway network usage has increased by 53 % in the last 20 years1. The amount of traffic per km of track is one of the highest worldwide, approaching 100 vehicle-km per km, per day, roughly double the amount of most other European railway networks2. In current international practice, the monitoring of the condition and the corresponding planning of maintenance of the infrastructure almost exclusively relies on on-site or workshop inspections, where specialized Non Destructive Evaluation methods (such as ultrasonics) are employed. Such inspections are either carried out in regularly spaced intervals or are prompted by the onset of irregular or potentially unsafe behavior. The leveraging of data that is extracted from instrumented vehicles allows to complement such schemes with an inflow of information, of diverse resolutions, that is related to condition. In Switzerland, the practice of gathering condition-relevant data via use of instrumented vehicles has enjoyed early adoption by the Swiss Federal Railways (SBB). The goal of OMISM was to exploit such data with the non-trivial aim of shifting the global condition assessment and monitoring paradigm for railways.
To this end, the team exploited a mix of on-board monitoring data obtained from both a diagnostic vehicle (gDFZ), as well as more continuous (but lower resolution) data from an instrumented in-service train (ICN) for diagnosing the health status and possible faults in railway infrastructure.
The project was structured in two interlaced tracks:
- Determine what quantities need be measured, i.e., which data is needed, complemented with mathematical/statistical models of the interacting system (Pillar I),
- Determine the processes and critical parameters that enable reduction in life cycle costs and performance improvement of the railway transport system (Pillar II).
Main outcomes and conclusions of the project
A new approach to monitoring the railway track relying on use of vibration data for deriving associated indicators of condition
In Pillar I, led by Cyprien Hoelzl under the guidance of Eleni Chatzi, Professor of Structural Mechanics and Monitoring at ETH Zurich, a comprehensive approach to monitoring the railway track was developed by primarily relying on exploitation of vibration-based data, and assessing their potency in terms of discovering long (longitudinal level) and short wavelength defects (surface defects, welds, squats).
Through applications in several case studies, we show the potential in adopting acceleration-based approaches for complementing and supporting traditional monitoring schemes. A data-driven approach, relying on a Vold-Kalman filter was introduced for identifying the periodic speed-dependent components of a measured axle box acceleration signal, such as the sleeper passage frequency and the wheel Out-Of-Roundness order. The wheel Out-Of-Roundness components are useful for detection of wheel defects, which is a side-outcome of the project relating more to the condition of the rolling stock, while the amplitude of the sleeper passage frequency is shown to form a proxy of the track condition (stiffness). Study on a track section from which on board monitoring data has been obtained, has further shown that track sections corresponding to higher stiffness seem to correlate to higher forces and increased maintenance actions.
The work further delves into assessment of critical components of the rail in what is termed a short-wave classification scheme. The rail forms an element whose structural integrity is essential for safety of operation, which is exposed to elevated loading that leads to frequent appearance of faults such as squats, cracks, or surface defects. An acceleration-based automated classification scheme is proposed for classifying main rail components and surface faults (healthy rail, surface defects and squats, insulated joints, and welds). Random Forests (RFs), which have been trained on engineered features, are contrasted against Convolutional Neural Networks (CNNs), which are trained on the Short Time Fourier Transform coefficients of the measured acceleration signals. While the validation of the scheme cannot be fully corroborated due to the difficulty in deriving labels, the implementation endorses automation and motivates implementation of acceleration-based classifiers to support early detection of faults.
The influence of reliability of labels from the available condition monitoring databases and the incorporation of expert feedback into the analysis process closes the loop of our suggested scheme. Concentrating on welds, as critical rail infrastructure components, we conducted a Proof-of-Concept study which brings OBM-derived indicators, database information and expert feedback in the loop. The study was conducted in collaboration with asset managers and field experts of the SBB. The framework combines on-site and visual inspections, diagnostic information, and expert assessments to automate the detection of weld defects from on board monitoring indicator outliers. The results of this first investigation are encouraging, but further reveal challenges such as those reflected in low precision and recall rates, which relate among others to the high uncertainty in the labels; an aspect which motivates further work.
Use of On-Board Monitoring data for effective decision-making in railway maintenance
Pillar II, led by Tzu-Hao Yan under the supervision of Francesco Corman, Professor of Transport Systems, initially focused on the principles for selecting and developing Track Quality Indices (TQIs) to gauge track conditions, aiming to facilitate the development of new TQIs based on emerging data types. Subsequent efforts involved proposing several track status degradation models, both novel and previously applied, utilizing On-Board Monitoring (OBM) data, meaning data acquired from sensors that are mounted on railway vehicles (both diagnostic and in service). This endeavor highlighted the models' respective strengths and limitations, such as resilience to irregular monitoring intervals or high sensor error rates, and examined the prediction accuracy of these models. A subsequent verification study demonstrated the feasibility of employing OBM data for scheduling efficient maintenance operations. Through comparative analyses, differences and similarities between the OBM and Track Recording Vehicle datasets were elucidated, alongside an assessment of the relationship between prediction accuracy, sensor error rates, and monitoring frequencies. Further research explored the Drivers' Response Failures (DRFs)—irregularities in track geometry identified by professional train operators—and their correlation with track conditions, aiming to assist rail infrastructure managers in formulating maintenance schedules. A maintenance and inspection scheduling model that integrates OBM inspections and DRFs was proposed, with case studies underscoring the benefits of this approach.
The insights garnered from both pillars were coupled into a comprehensive framework by leveraging condition indicators derived in Pillar I and integrating these into Pillar II to optimize maintenance planning policies.
The way forward
Looking ahead, Pillar I intends to explore additional elements such as bridge structures and switch components, which are pivotal components of the network that could benefit significantly from vehicle-based condition assessment. Despite the rising popularity of on-site sensor monitoring for these assets, vehicle-based surveillance promises greater scalability. Pillar II aims to delve deeper into the application of OBM inspection data in maintenance and inspection scheduling, posing a pertinent research question: What is the minimum number of commercial trains required to be equipped with OBM techniques to attain a satisfactory level of system understanding without incurring excessive costs? Given the constraints of sensor installation and removal at depots, alongside timetable and rolling stock scheduling constraints, this model seeks to identify the optimal number of trains for OBM technique deployment, ensuring sufficient data coverage across different rail sections.
-
Professor Eleni Chatzi (Institute of Structural Engineering, ETH Zurich) -
Professor Francesco Corman (Institute for Transport Planning and Systems, ETH Zurich) -
Dr. Cyprien Hoelzl (Institute of Structural Engineering, ETH Zurich) -
Dr. Tzu-Hao Yan (Institute for Transport Planning and Systems, ETH Zurich)
Reflecting on their collaboration, Eleni Chatzi and Cyprien Hoelzl acknowledge the challenges encountered, which relate to application within a practical setting of elevated requirements. The partnership with SBB proved instrumental in revealing challenges and effectuating an approach relying on fusion of diverse information for opening up new pathways for research and implementation.
Similarly, Francesco Corman and Tzu-Hao Yan highlight the challenges posed by low-quality or unavailable datasets, exacerbated by the mismatch between academic and industry timelines. The availability of real-world data, however, validated their research efforts. SBB's collaboration not only furnished essential data but also facilitated an understanding of practical maintenance and inspection scheduling processes, enabling Tzu-Hao to propose a more viable scheduling model. The frequent interactions prevented redundant studies, allowing both parties to focus on their primary objectives more effectively.
Thomas Wissert from SBB lauds the smooth collaboration, initially hampered by COVID-19, and acknowledges the project's technical feasibility and the importance of data quality for asset monitoring. SBB appreciates the autonomy granted to students, resulting in beneficial outcomes, and expresses a willingness to engage in future innovative projects with ETH.

“When venturing into innovation projects, where the theoretical and innovative content is key, I would be very happy to collaborate again with ETH. It allows us to benefit from the quality of the technical research.”Thomas Wissart (SBB)![]()
The project partner would like to additionally thank, in particular, to the Metrology (MUD) and Strategic Asset Management of the Track (SAFB) departments, including Aurelia Kollros, Marcel Zurkirchen, Lucian Ancu and Stanislaw Banaszak, as well as the track experts of the SBB, who supported the development of this Proof-of-Concept dataset of defect welds.